Statistical Genetics
TAMU Collaborators
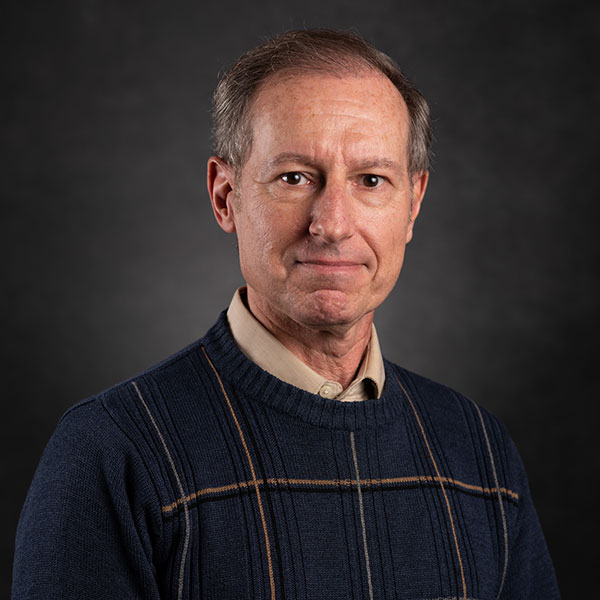
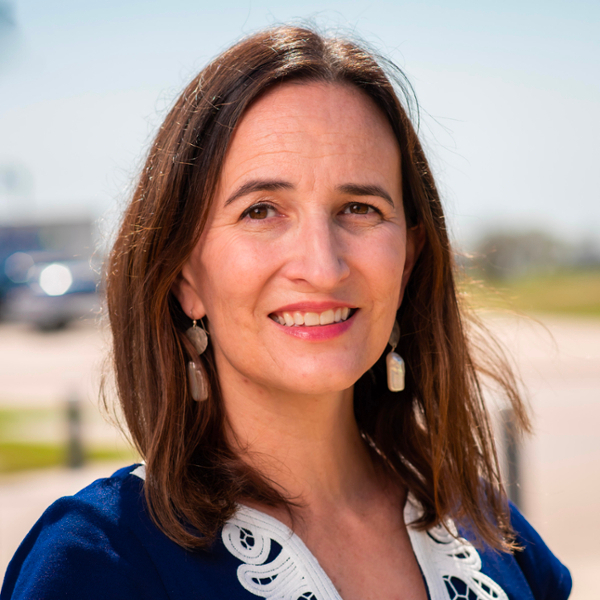
Outside Collaborators
- Dr. Michael Neale, Ph.D. (Virginia Commonwealth University)
- Dr. Joshua Pritikin, Ph.D. (Virginia Commonwealth University)
- Dr. Elizabeth Prom-Wormley, Ph.D. (Virginia Commonwealth University)
Contact Us
If you are interested in more information please email Dr. Verhulst@tamu.edu